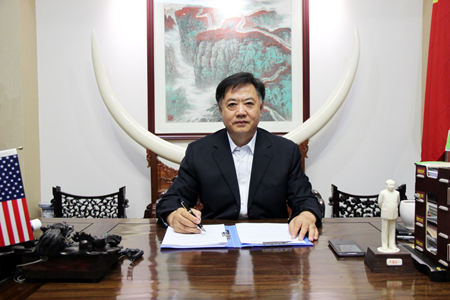
CCTV News:For any country in the world, the fate of manufacturing industry has a profound impact on the overall appearance and process of social and economic development.
With the arrival of the new normal of China’s economy, China’s manufacturing industry has been in a dilemma for a time: on the one hand, nearly 1.4 billion people have brought a huge consumer market; On the other hand, it is difficult for China’s manufacturing industry to produce high-end products with market competitiveness, such as Japanese toilet seats, Australian milk powder and Korean cosmetics … … Consumers have flocked abroad to sweep the goods.
In the past 30 years, China’s manufacturing industry has developed rapidly and on a large scale, but the overall quality is not high. Short-term demand can be met, but long-term supply capacity is not enough. With the low-cost advantage of China’s economic development and the gradual disappearance of demographic dividend, as well as the rapid development of e-commerce economy, China’s manufacturing industry has encountered the double attack of weak endogenous growth and external Internet impact, and the supply-side reform, transformation and upgrading of manufacturing industry are waiting for us.
In Hai ‘an, Jiangsu Province, a representative manufacturing enterprise that upholds the spirit of artisans and has been deeply involved in manufacturing for 30 years — — Haixun Group, under the leadership of "parent" Zhong Jihe, keeps pace with the times and is determined to reform, transform and upgrade, which is a model for the exploration of supply-side reform in manufacturing industry. A few days ago, with the opportunity of its 30th anniversary, Haixun Group, Nantong Daily, Hai ‘an County Development and Reform Commission and Xinhuanet Jiangsu jointly held the "Supply-side Reform and Craftsman Spirit Summit Forum and the 30th Anniversary Celebration of Haixun Group", which shared its own experience and thinking in the transformation and upgrading of manufacturing industry. It was full of dry goods and inspired a lot.
When talking about the experience and original intention of reform, Zhong Jihe, chairman of Haixun Group, said: Haixun has kept the initial intention of manufacturing enterprises for 30 years, constantly innovating manufacturing processes, improving manufacturing standards and upgrading manufacturing technology and knowledge with the spirit of artisans; At the same time, the development of manufacturing industry should be good at fake things, stay young, use internet tools to improve manufacturing efficiency, improve technology and stimulate innovation; In addition, Haixun relies on the power of finance to incite transformation and upgrading, take advantage of the trend, integrate industrial and financial resources, and build a business incubation platform with capital as a link.
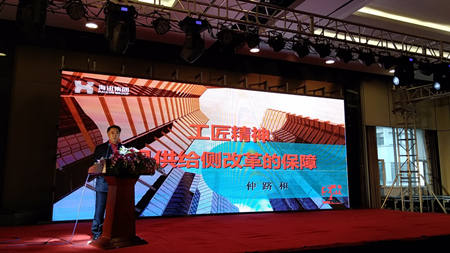
Work hard and be determined to reform
Zhong Jihe was a veteran, joined the army in 1978, served as monitor and acting platoon leader, and was awarded seven times in five years. After being admitted to a military academy, he returned to his hometown due to illness. For the sake of living, he opened a small shop and did a small business of providing oil, salt, sauce and vinegar services for his neighbors. In 1986, he first entered the then No.3 Rubber Factory in Hai ‘an County (predecessor of Haixun Group) as a supply and marketing clerk. At that time, there were only a dozen workers in the rubber factory, with an annual output value of several hundred thousand yuan, and the profit was always "carrying a pole" — — Loss. Six months after entering the factory, Zhong Jihe became the director of the rubber factory and took on the responsibility of "supporting the family". In order to save costs, he personally delivered the goods and unloaded the goods. In the meantime, in order to let customers know more about their products, so as to enter the elevator industry and turn losses into profits, he once walked from Lugouqiao for four hours to Shen’s customer’s home in Beijing, waited outside the door for more than an hour, and finally impressed customers with sincerity and interpreted a story of "Shen Men Li Shuang".
Regarding reform, Zhong Jihe said: "The so-called transformation and upgrading, as well as the supply-side reform that everyone is talking about, is actually a constant self-denial. Only by constantly learning can we constantly deny ourselves. Only by constantly self-denying, enterprises will have innovation. "
In the development of Haixun, Zhong Jihe led four extremely important "self-denial": for the first time, in 1992, he was the first in the county to implement the reform of joint-stock cooperative system, so that employees could truly become the owners of enterprises; The second time, in 1994, a joint venture with China Schindler entered the elevator accessories industry, which enabled the enterprise to enter the fast lane of development; The third time, in 1997, the equity transfer was implemented, and the nature of the enterprise changed to private ownership; For the fourth time, in 2004, Nantong Haixun Treca Elevator Products Co., Ltd. was established in a joint venture with Treca, USA, laying a solid foundation for elevator accessories products to enter the world market.
In 1992, Zhongjihe took the lead in carrying out the reform of the joint-stock cooperative system. The new mechanism worked well at first, but soon the problem came: because the government and social legal persons did not understand the enterprise and the market, holding the board meeting not only delayed the decision-making of the enterprise, but also missed the opportunity when implementing it. After discovering the defects of the joint-stock system, Zhong Jihe made up his mind to acquire government shares and social legal person shares through equity transfer and set up a real private enterprise. This is to deny yourself in the operation mechanism of the enterprise.
Haixun has also found development breakthroughs in the market and products through continuous denial. From the beginning to make bicycle accessories and leather shoes soles, to make triangle belts and elevator accessories later; However, when the sales situation of elevator accessories was very good, it was found that the market for this kind of products was small, so it was transformed to produce railway equipment, and then entered the fields of nano-new materials and ecological energy. All these are of great strategic significance to Haixun’s industrial innovation.
In addition, we constantly deny ourselves in management. With the development of the enterprise, Zhong Jihe has continuously entered famous universities such as Tsinghua, Peking University, People’s Congress, Fudan University and Nanda University, including Taiwan Province University, and integrated his study into his daily life and work, learning with the problems of the enterprise, and applying the learned knowledge to enterprise management in time.
Under the leadership of Zhongjihe, the enterprise has carried out step-by-step operations such as merger, cooperation and joint venture, and established a series of wholly-owned, holding and shareholding subsidiaries, eventually forming a group operation mechanism. In 30 years, Zhongjihe developed a small factory from a hometown into a large group enterprise involved in elevator parts, railway equipment, financial services, agricultural services and ecological energy by relying on technological innovation and management innovation. Today, Haixun has developed into a large industrial group with total assets of more than 2 billion yuan and more than 1,000 employees, with branches in Beijing, Hunan, Hubei, Anhui, Guangdong and Guangxi.
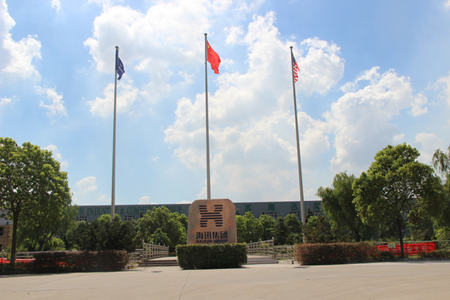
Scientific and technological innovation, talent-oriented
Thirty years ago, Haixun, a humble factory building, and a rubber vulcanizer heated by coal were backward in technology and hard to say. The office is less than 30 square meters, and several people share a desk, so the business operation is struggling. However, in the subsequent development, thanks to Zhongjihe’s spirit of reform and innovation, Haixun continued to enter the emerging market field by relying on scientific and technological innovation.
In 1994, Zhongjie entered the elevator accessories industry and made a joint venture with China Schindler Elevator to promote Haixun to enter the elevator industry; In 2004, Haixun established a joint venture with Treca Company, a world-renowned elevator company, which opened the way for elevator accessories to enter the international market. In 2005, we began to explore Beijing and Tianjin markets, and at the same time cooperated with Hebei University of Technology to enter the field of nano-materials. In 2007, the Railway Equipment Group was established, with "making railway equipment bigger" as the strategic focus. The enterprise has successively won a number of manufacturing licenses, becoming one of the enterprises with the most CCRC certification and manufacturing licenses in the railway parts industry; In 2010, Haixun expanded its factory in Hai ‘an Development Zone and started the production project of complete rail transit system. In 2014, he entered the power industry and became a shareholder in Leon Ecological Energy Co., Ltd..
Scientific and technological innovation is based on talents. Zhong Jihe has a point of view: enterprises are raising depreciation expenses every year, but the real depreciation is people, not their knowledge. To this end, Zhong Jihe has jointly organized "training courses" with Shanghai Fudan School of Management and Nanda Business School; At the same time, with the help of external brain to help scientific and technological innovation, we have successively carried out Industry-University-Research activities with Qingdao University of Science and Technology, Hebei University of Technology, Shanghai Jiaotong University and Tianjin University, which not only realized the landing of scientific research achievements, but also enhanced Haixun’s core scientific and technological competitiveness.
In the tide of Industry 4.0, Haixun Group increased R&D investment, pursued scientific and technological innovation, and completed the transformation and upgrading of the group from components to end products through technical transformation and project grafting. "internet plus Railway Equipment" and "internet plus Elevator Parts" participate in the "Belt and Road", integrate into the construction of smart cities, and expand sales; Replace people with machines, fully implement automation transformation and digital factory construction, set up enterprise research institutes, and carry out in-depth cooperation in Industry-University-Research.
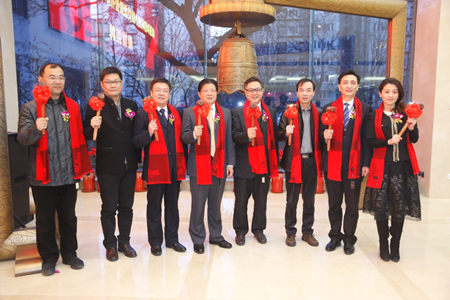
Governing enterprises with Confucianism is deeply rooted in morality.
Industry, agriculture, commerce, learning, and the military have rich experience. Although they are from the military, they are full of literati in their bones. They are especially good at reading and writing after management. He covers a wide range of fields, including literature, history, philosophy, Confucianism, Taoism, Buddhism, biographies, education, business management and so on, with a personal collection of more than 5,000 volumes, and is especially fond of classical works such as Confucius, Mencius and Zhuangzi. In addition, in order to enrich employees’ spare-time cultural life, he also cooperated with Hai ‘an County Library to establish "Haixun Library", providing more than 3,000 books for employees to borrow.
After reading, Zhong Jihe is diligent in writing. He has published nearly 200 essays in Yuhua, Jiangsu Prose, Jianghai Evening News, Yuedu and other newspapers and periodicals, and successively published essays such as Dreams Fly, Men’s Feelings, Flowers Smile, Unintentionally Leaving Traces, Dancing with the Wind, and Enterprise Management Anthology, Career Answers. His book "Building a Cultural System Based on Enhancing the Core Competitiveness of Enterprises" won the second prize in the paper appraisal of "Enterprise Reform and Management" in Jiangsu Province. Because of his fruitful literary creation, he joined Jiangsu Writers Association and China Prose Society, and was elected as the vice chairman of Hai ‘an Writers Association and Nantong Writers Association, and joined Jiangsu Writers Association, China Prose Society and Deputy Secretary General of Jiangsu Prose Society.
Zhong Jihe attaches great importance to drawing nutrition from traditional culture. "The most influential factor in corporate culture is people, people based on historical and cultural traditions, people based on the development of industrial enterprises, and people based on the trend of social times." Talent is the foundation of enterprise development, and enterprise cohesion depends on enterprise culture. Enterprise development "depends not only on hardship, technology, equipment, market and opportunity, but also on enterprise culture".
"Culture is a value, and values are a ruler. If you don’t have a common language, you can’t have a common standard for judging things if you speak with a different accent. With corporate culture, employees have a common standard for judging right and wrong. " In this regard, Zhong Jihe launched employee discussions at the beginning of the factory to refine the entrepreneurial spirit — — "Family culture". Inspired by this, Haixun Group has formed a corporate culture atmosphere of hard-working, hard struggle, prohibition and vigorous action, keeping pace with the times, paying attention to learning, taking the lead by leaders and dedicating all staff in the course of 30 years’ development.
Haixun’s "home" culture is not only a signboard, but also a golden key to unite people and go to the market. Its purpose is to let employees think of responsibility when they see "home"; Let customers feel warm when they see "home" This is the most basic core of the spirit of "home".
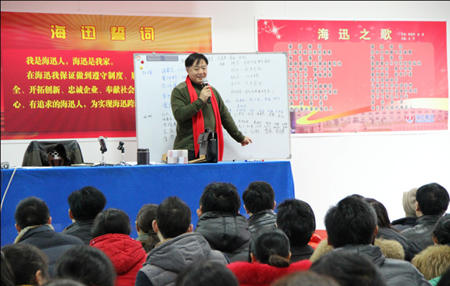
Craftsman spirit, precision work and wisdom.
Zhong Jihe said: "If you are too impetuous, you can’t do a good job in the manufacturing industry. The manufacturing industry needs innovation, but you must know how to be clumsy. Haixun will continue to be a craftsman in the manufacturing industry." "Many companies want to transform, but they don’t know where to turn. My experience is that if you consider doing something, firstly, it is worth doing, secondly, someone will do it, and thirdly, I have the ability to do it. Only when you have all three can you do it, and you can do it without one. Haixun has been doing what he is familiar with in recent years. Such as real estate, we have never touched it. Whether it is diversification now or transformation to large agriculture in the future, it is still connected internally. " "I still hope to be able to do things in a down-to-earth manner and do a good job in the long run. Do what you can, and you won’t regret it in life. "
Strictly demanding and consistent on quality; Be brave in exploring and actively improving technological innovation. The craftsman spirit flows in the blood of every Haixun person. Craftsman spirit is to focus on all aspects of production with the attitude of craftsman, and to pursue perfection and Excellence in quality. This is the cultural guarantee for the success of supply-side reform.
Thanks to the creative team with innovative consciousness and perseverance, Haixun Group has successively won 25 patents for utility models and 16 patents for inventions, among which dozens of products have been listed as national torch plan, excellent new products, high-tech products in Jiangsu Province and key new products in Jiangsu Province. In 2016, Haixun Group continued to promote industrial upgrading and transformation and actively adjusted its corporate strategy; Through multidimensional integration, the industrial layout involves six major sectors, including railways, elevators, chemicals, finance, ecological energy and agricultural machinery. Zhong Jihe, chairman of Haixun Group, said: In the future, Haixun will focus on the diversified development of "craftsman Haixun, technology Haixun, industry and finance Haixun, and ecological Haixun". Relying on the blessing of financial power, Haixun in the future will become an incubator platform for listed companies and build an industrial cluster of Haixun. We expect Haixun Group to create brilliant achievements in the next 30 years. Hai Xun starts again, Hai Xun is on his way.